What is the Future Of Artificial Intelligence?
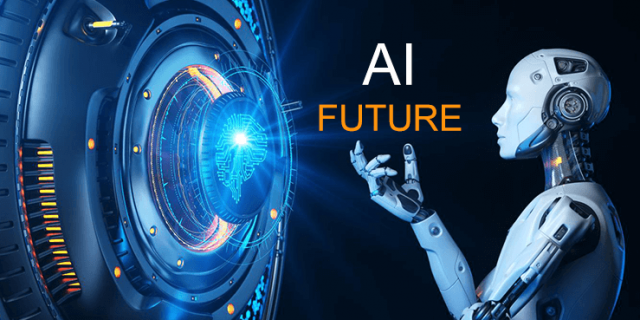
Large technological companies like Google, Facebook, and Microsoft are already making investments in the scalability of AI in a variety of commercial fields.
Published: 2023-04-03
According to the article of Manas Agrawal for Forbes, by 2030, AI use is projected to move beyond straightforward use cases and applications. It will be required to anticipate weather patterns over a wide region for several months, identify life-threatening illnesses in their early stages, and work alongside humans digitally. These are just a few examples of how AI may affect daily life and the workplace in the years to come. In the industry, the rate of change has been unparalleled, and it looks like it will stay that way in the years to come.
AI is no longer a futuristic technology because of its quick learning and acceptance; instead, it is a part of practically every aspect of human existence. In fact, the changes brought on by AI are now so prevalent that they have a significant impact on user experience and how people engage with products and technology. Given the current course of events, AI will quickly integrate itself into daily life and civilization.
The fact that AI is continuously evolving will contribute to its widespread acceptance and a wide range of new application cases. It already achieves quicker calculation, more accuracy, and reduced infrastructure and processing expenses. The fact that AI is now developing in all three of these areas — compute data, and algorithm — creates the conditions for its widespread acceptance in all spheres of life and work by 2030. Here is how I envision AI developing in various fields.
Compute
The easiest to quantify of all the main forces influencing the growth of AI is computation. The computing industry will undergo a significant change in the upcoming ten years. Application-Specific Integrated Circuits (ASIC) and Field Programmable Gate Arrays are replacing graphic processing units (GPU) (FPGA). This is so because the performance of ASIC and FPGA is superior to that of GPU.
ASIC will employ multicore processing to do sophisticated AI tasks while using less energy. The tensor processing unit, an ASIC created for the cloud, is one example of how ASIC is becoming so prevalent that Google has invested in its construction.
FPGA will go things further by enabling designers to rearrange designer pieces. The fact that Amazon invested in FPGA through AWS Inferential is evidence that FPGA will genuinely revolutionize the computing component of AI over the next ten years. IPUs (intelligent processing units), which concentrate on huge parallelization of complicated high dimensional models and offer high compute density, will also undergo significant change. These are all indications that the compute aspect of AI is undergoing a fundamental transition that will continue over the next ten years.
Data
AI's data component will change in terms of the number of sources, amount of information, and processing technique. Future processing of increasingly complicated interactions will require additional sources from IoT, greater information from data captured every millisecond, and multi-modal data intake by DL approaches. As data scientists need to acquire datasets affordably and impart their analysis to deep learning (DL) models, data plays a crucial role in the advancement of AI.
The way AI uses data to generate precise predictions has already undergone something of a revolution. Digital sand is being produced by sensors and IoT devices. Data is being generated via logs from impact systems with millisecond response times. People are producing data through conventional touchpoints and systems that produce zettabytes of data during fundamental physical processes (such as a chemical reaction), which combined are poised to change how AI infers data. The new norm now involves drawing conclusions about data from unexpected sources.
These factors point to an inevitable revolution in the data aspect of AI that will occur over the next ten years.
Algorithm
The way an artificial intelligence (AI) thinks about a scenario might be somewhat similar to how a person would see the same situation with future developments in artificial neural networks (ANN). This is significant because it would allow us to develop DL models, which can do accurate analysis even with little data.
New algorithms that are geared at managing complex data, speed, parallel computing, cost, accuracy, and precision are being created every single day. In few shots learning, for instance, the emphasis is on learning more and deeper while using less labeled data sets. In order to ease the parallelization of tensor processing and speed up computation, Distributed DL is developing a collection of methods. Almost all NLP tasks can be solved by GPT3 with the maximum level of accuracy. In computer vision, experts are using the idea of transformers to make algorithms more context-aware, which saves time by eliminating the need to train pictures in all conceivable orientations. Unsupervised domain-free anomaly detection is accomplished using variational auto-encoders.
Also, there is a stronger focus on reinforcement learning, with inductive learning strategies like model-free learning being used. Better learning is possible in a multi-agent system thanks to a parallel training framework. As a result, highly effective collaborative robot systems will be produced. These new advances will all be incorporated into the algorithm component's overall metamorphosis.
Conclusion
Large technological companies like Google, Facebook, and Microsoft are already making investments in the scalability of AI in a variety of commercial fields. It's expected that no significant vertical will be unaffected by AI by the year 2030. The technology is well on its way to providing a larger reach, being much faster, and being so affordable that it will become a part of not only the everyday life of major corporations but also of every average man. AI will be a brand-new, widespread, and incredibly potent kind of mobile technology. The world we live in will be driven by AI, and I think the businesses that start planning for this change now will be the ones that succeed in ten years.
Those that get the significance of data, algorithms, and computational architectures and are capable of using the changes in these areas in genuinely effective ways will truly own the decade. AI will change many industries, and business leaders need to get ready for these breakthroughs. Observe this section.